SILO Language Models: Isolating Legal Risk In a Nonparametric Datastore
Abstract Commentary & Rating
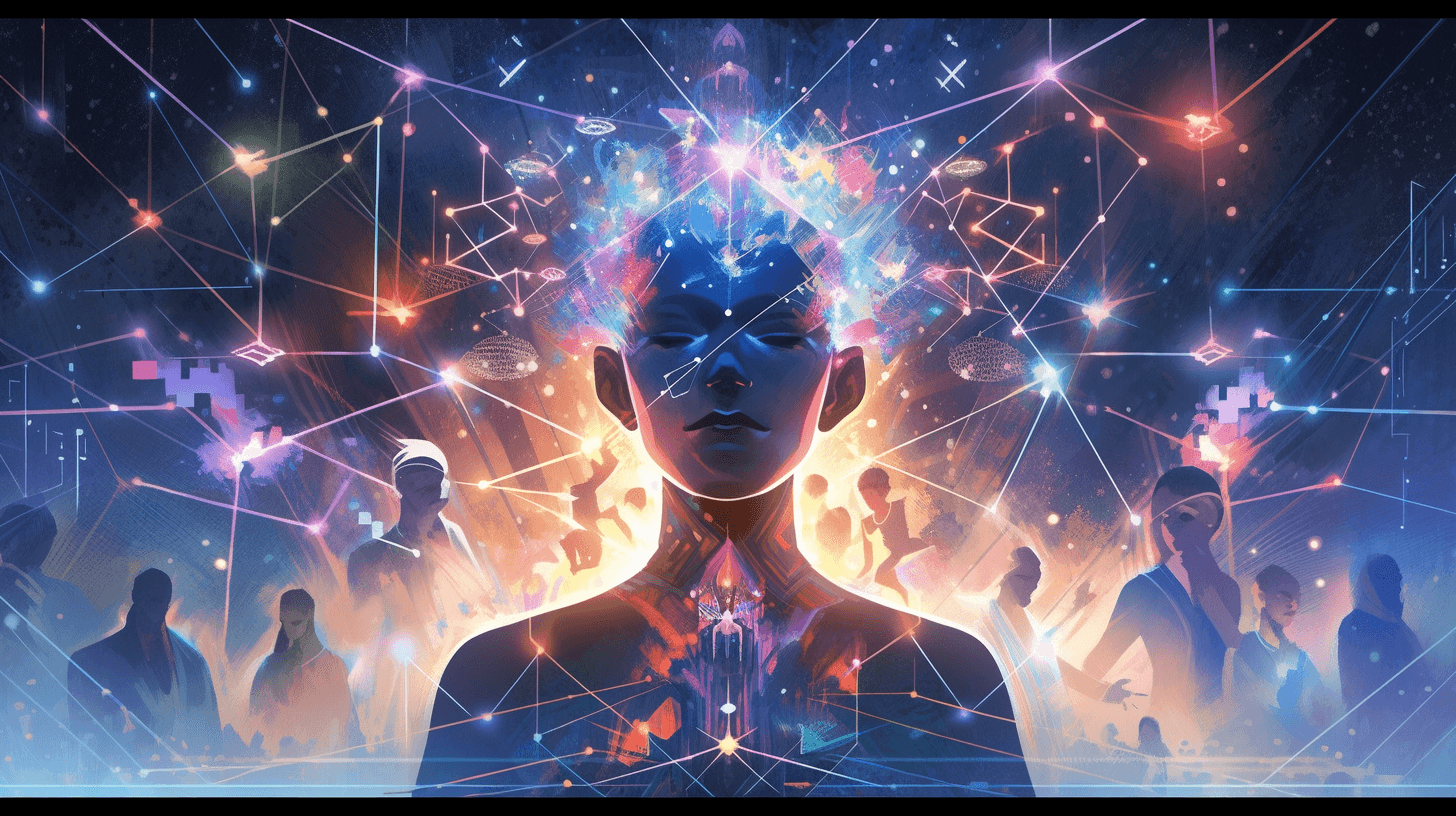
Published on Aug 8
Authors:Sewon Min,Suchin Gururangan,Eric Wallace,Hannaneh Hajishirzi,Noah A. Smith,Luke Zettlemoyer
Abstract
The legality of training language models (LMs) on copyrighted or otherwise restricted data is under intense debate. However, as we show, model performance significantly degrades if trained only on low-risk text (e.g., out-of-copyright books or government documents), due to its limited size and domain coverage. We present SILO, a new language model that manages this risk-performance tradeoff during inference. SILO is built by (1) training a parametric LM on Open License Corpus (OLC), a new corpus we curate with 228B tokens of public domain and permissively licensed text and (2) augmenting it with a more general and easily modifiable nonparametric datastore (e.g., containing copyrighted books or news) that is only queried during inference. The datastore allows use of high-risk data without training on it, supports sentence-level data attribution, and enables data producers to opt out from the model by removing content from the store. These capabilities can foster compliance with data-use regulations such as the fair use doctrine in the United States and the GDPR in the European Union. Our experiments show that the parametric LM struggles on domains not covered by OLC. However, access to the datastore greatly improves out of domain performance, closing 90% of the performance gap with an LM trained on the Pile, a more diverse corpus with mostly high-risk text. We also analyze which nonparametric approach works best, where the remaining errors lie, and how performance scales with datastore size. Our results suggest that it is possible to build high quality language models while mitigating their legal risk.
Commentary
The paper "SILO Language Models: Isolating Legal Risk In a Nonparametric Datastore" delves into a timely and relevant area of language model research, especially considering the increasing scrutiny over data rights, copyright issues, and broader ethical concerns tied to AI.
Significance:
Legal Implications: Addressing the legality of training models on copyrighted or restricted data is essential. With increasing concerns about data privacy and misuse, finding ways to operate within legal bounds becomes paramount.
Risk-Performance Tradeoff: The paper introduces SILO, which balances performance with the legal risk associated with data, presenting a solution that's both technically sound and legally compliant.
Nonparametric Datastore: By not training on high-risk data but using it only during inference, SILO presents a unique way to leverage vast information without infringing on copyright or other restrictions. This can be an innovative step forward in responsible AI modeling.
Data Attribution & Opt-out: The capacity for sentence-level data attribution and the option for content creators to remove their content helps further the cause of data rights and provides greater control over one's data.
Impact:
Legal Compliance: Companies and researchers can use such models to avoid potential legal challenges and adhere to data-use regulations, promoting the responsible use of AI.
Encouraging Open Licensing: The creation of the Open License Corpus might spur further efforts to create open, extensive datasets that can be used safely in model training.
Greater Trust: By addressing copyright concerns and offering a mechanism for data attribution and removal, this approach could foster greater public trust in AI systems.
Research Benchmarking: The techniques proposed can provide a reference for other research aiming to address similar concerns, thus potentially setting a benchmark in the domain.
Economic Implications: Avoiding potential lawsuits and promoting legally compliant AI can lead to savings and reduce the economic risk for businesses deploying AI models.
Considerations:
Implementation Complexity: The dual nature of the model (parametric plus nonparametric) may introduce complexities in terms of implementation and optimization.
Datastore Scalability: As the size of datastores grows, ensuring efficient querying and real-time inference could be challenging.
Given the importance of addressing legal risks, copyright concerns, and data rights in the world of AI, coupled with the innovative solutions proposed to address performance degradation when avoiding high-risk text, I'd rate the potential real-world impact of this paper as 9 out of 10. As AI applications become pervasive in society, ensuring they respect data rights and legal boundaries is crucial for sustainable growth.