Shepherd: A Critic for Language Model Generation
Abstract Commentary & Rating
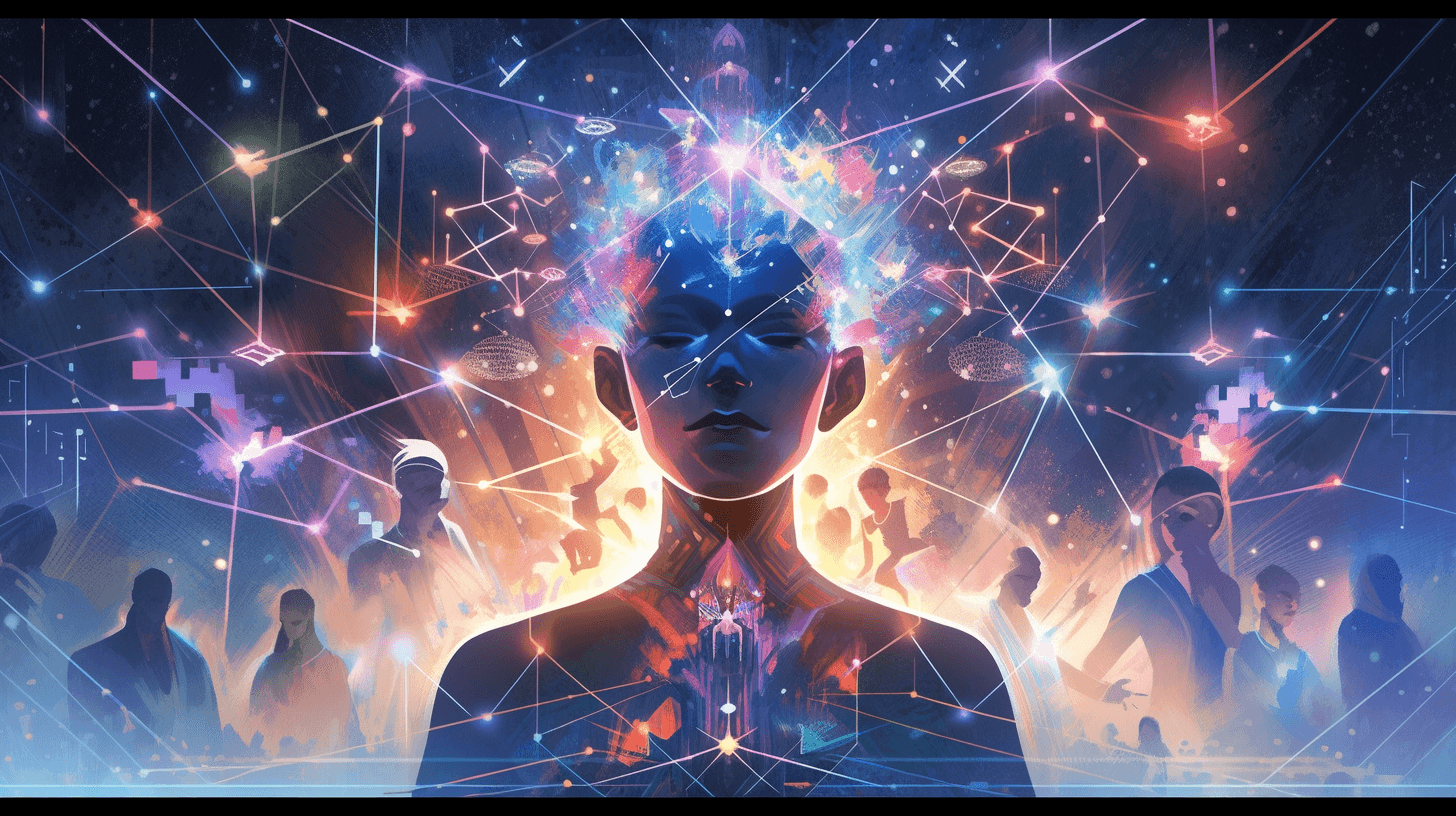
Published on Aug 8
Authors:Tianlu Wang,Ping Yu,Xiaoqing Ellen Tan,Sean O'Brien,Ramakanth Pasunuru,Jane Dwivedi-Yu,Olga Golovneva,Luke Zettlemoyer,Maryam Fazel-Zarandi,Asli Celikyilmaz
Abstract
As large language models improve, there is increasing interest in techniques that leverage these models' capabilities to refine their own outputs. In this work, we introduce Shepherd, a language model specifically tuned to critique responses and suggest refinements, extending beyond the capabilities of an untuned model to identify diverse errors and provide suggestions to remedy them. At the core of our approach is a high quality feedback dataset, which we curate from community feedback and human annotations. Even though Shepherd is small (7B parameters), its critiques are either equivalent or preferred to those from established models including ChatGPT. Using GPT-4 for evaluation, Shepherd reaches an average win-rate of 53-87% compared to competitive alternatives. In human evaluation, Shepherd strictly outperforms other models and on average closely ties with ChatGPT.
Commentary
The paper "Shepherd: A Critic for Language Model Generation" dives into the area of refining outputs produced by large language models. This domain is crucial as the next step in AI research is not just producing answers but ensuring that these answers are of the highest quality.
Significance:
Refining Outputs: As the usage of large language models becomes more widespread, the quality and reliability of their outputs become paramount. Shepherd offers a way to evaluate and refine these outputs, which can significantly improve the end-user experience.
High-Quality Feedback Dataset: The use of a dataset curated from community feedback and human annotations means that Shepherd's critiques are based on real-world feedback, which may be more representative of general user concerns and issues.
Size vs. Performance: Shepherd's relatively small size (7B parameters) but comparable or even superior performance to larger models demonstrates that, with the right training and dataset, smaller models can still achieve high performance.
Impact:
Improved AI Responses: Deploying Shepherd alongside large language models can help in dynamically refining model responses, making them more accurate, relevant, and contextually appropriate.
Human-AI Collaboration: By offering critiques, Shepherd can act as a bridge between the AI's initial response and the final output, allowing human users to make informed decisions based on these critiques.
Efficiency: For applications where resources are constrained, Shepherd's smaller size but robust performance can be a game-changer.
Educational Applications: For platforms leveraging AI for educational purposes, Shepherd can be instrumental in ensuring that learners receive accurate and high-quality responses.
Model Debugging and Refinement: Developers and researchers can use Shepherd's critiques to understand areas of improvement in their models and iteratively refine them.
Considerations:
Dataset Limitations: While community feedback and human annotations are valuable, there's a possibility that this dataset might have its own biases or might not be comprehensive.
Model Acceptance: Users and developers need to trust and accept Shepherd's critiques for it to have a real-world impact. This acceptance will come with demonstrations of its efficacy.
Given the increasing reliance on large language models and the importance of high-quality outputs in real-world applications, combined with Shepherd's promising approach to critique and refine these outputs, I'd rate the potential real-world impact of this paper as 8.5 out of 10. Ensuring the quality of AI-generated content is crucial, and Shepherd appears to offer a promising avenue towards achieving this goal.