Clinical Text Summarization: Adapting Large Language Models Can Outperform Human Experts
Abstract Commentary & Rating
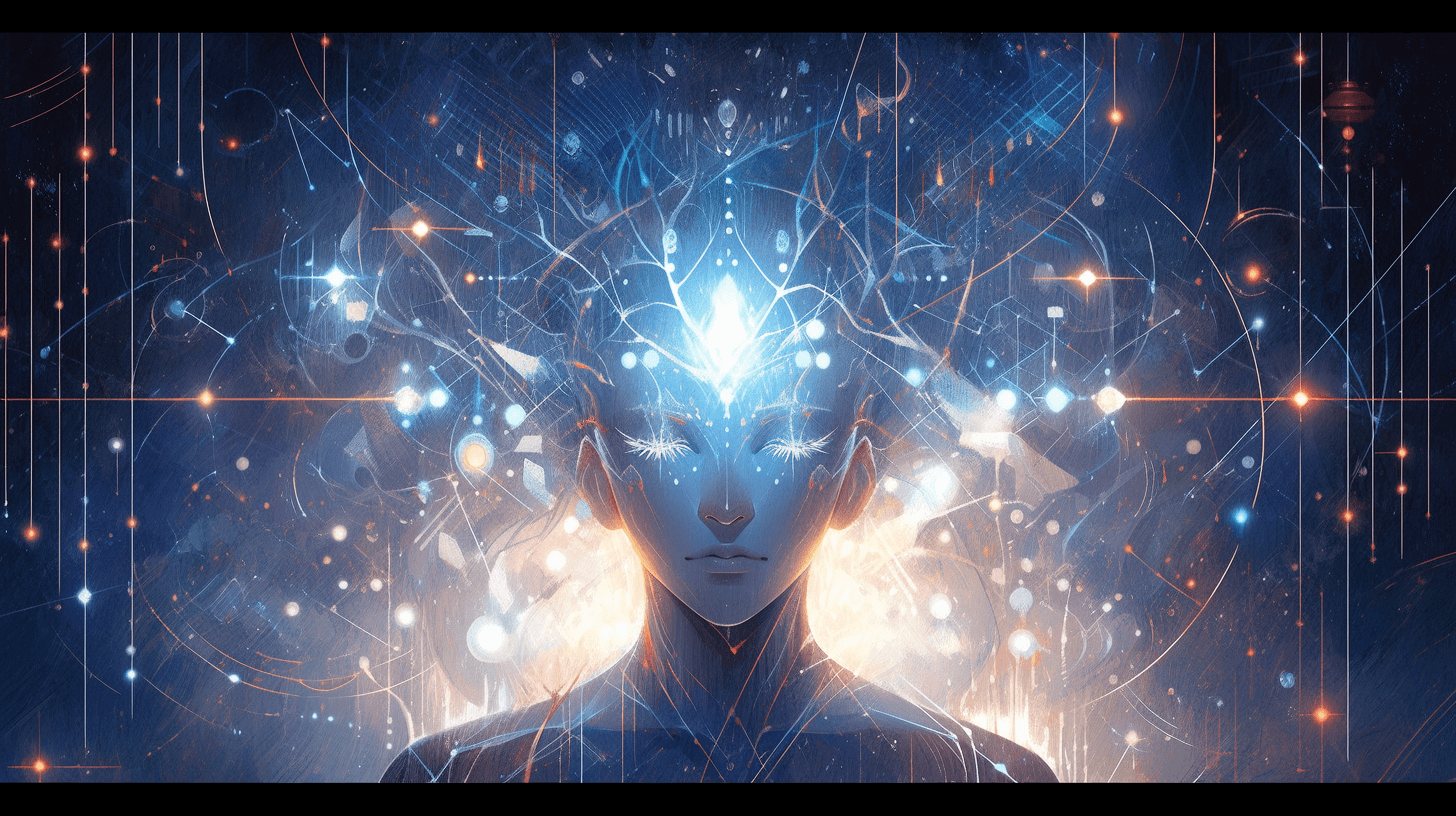
Published on Sep 13
Authors:Dave Van Veen,Cara Van Uden,Louis Blankemeier,Jean-Benoit Delbrouck,Asad Aali,Christian Bluethgen,Anuj Pareek,Malgorzata Polacin,William Collins,Neera Ahuja,Curtis P. Langlotz,Jason Hom,Sergios Gatidis,John Pauly,Akshay S. Chaudhari
Abstract
Sifting through vast textual data and summarizing key information imposes a substantial burden on how clinicians allocate their time. Although large language models (LLMs) have shown immense promise in natural language processing (NLP) tasks, their efficacy across diverse clinical summarization tasks has not yet been rigorously examined. In this work, we employ domain adaptation methods on eight LLMs, spanning six datasets and four distinct summarization tasks: radiology reports, patient questions, progress notes, and doctor-patient dialogue. Our thorough quantitative assessment reveals trade-offs between models and adaptation methods in addition to instances where recent advances in LLMs may not lead to improved results. Further, in a clinical reader study with six physicians, we depict that summaries from the best adapted LLM are preferable to human summaries in terms of completeness and correctness. Our ensuing qualitative analysis delineates mutual challenges faced by both LLMs and human experts. Lastly, we correlate traditional quantitative NLP metrics with reader study scores to enhance our understanding of how these metrics align with physician preferences. Our research marks the first evidence of LLMs outperforming human experts in clinical text summarization across multiple tasks. This implies that integrating LLMs into clinical workflows could alleviate documentation burden, empowering clinicians to focus more on personalized patient care and other irreplaceable human aspects of medicine.
Commentary
The paper titled "Clinical Text Summarization: Adapting Large Language Models Can Outperform Human Experts" delves into a significant application area of Large Language Models (LLMs) – clinical text summarization.
Key Takeaways:
Clinical Importance: The paper addresses the critical challenge of sifting through vast clinical textual data, which consumes a significant amount of clinicians' time.
Diverse Application: The paper explores LLMs' efficacy in summarizing four distinct types of clinical texts: radiology reports, patient questions, progress notes, and doctor-patient dialogue.
Superiority to Human Summaries: Notably, in certain tasks, LLM-generated summaries were found preferable to human-generated ones in terms of completeness and correctness.
In-depth Analysis: The research offers both a quantitative assessment of the models and a qualitative analysis that highlights challenges faced by both humans and LLMs in this domain.
Real-World Impact:
Time-saving for Clinicians: If integrated into clinical workflows, such a tool can significantly reduce the time doctors spend on documentation, allowing them more time for actual patient care.
Improved Patient Care: By enabling more concise and accurate summaries of patient information, clinicians might make more informed decisions, potentially leading to better patient outcomes.
Educational Value: For medical students and junior doctors, such summaries can serve as effective learning aids, especially when dealing with unfamiliar cases.
Inter-departmental Communication: Accurate summarization can aid smoother communication between different departments in a hospital, such as between radiology and surgery.
EHR Integration: Integration of this tool into Electronic Health Records (EHR) systems can make EHRs more user-friendly and actionable.
Challenges and considerations:
Dependability: Given the critical nature of medical data, the reliability of such summaries will be paramount. A single oversight can have significant clinical consequences.
Data Privacy: Handling patient data brings along significant privacy and ethical considerations. Ensuring the protection of patient data will be crucial.
Generalizability: It remains to be seen how well such models perform across diverse patient populations and different healthcare systems.
Given the significant time and accuracy challenges clinicians face with documentation, combined with the paper's findings that LLMs can outperform human experts:
I'd rate the real-world impact of this paper as a 10 out of 10.
The potential to transform clinical documentation processes, improve inter-departmental communication, and ultimately enhance patient care makes the findings of this paper highly impactful. However, the real-world implementation will require careful consideration of data privacy and model reliability.