Open Problems and Fundamental Limitations of Reinforcement Learning from Human Feedback [Summary]
Uncover the challenges, limitations, and future of Reinforcement Learning from Human Feedback (RLHF) in AI systems. Explore governance, safety, and more.
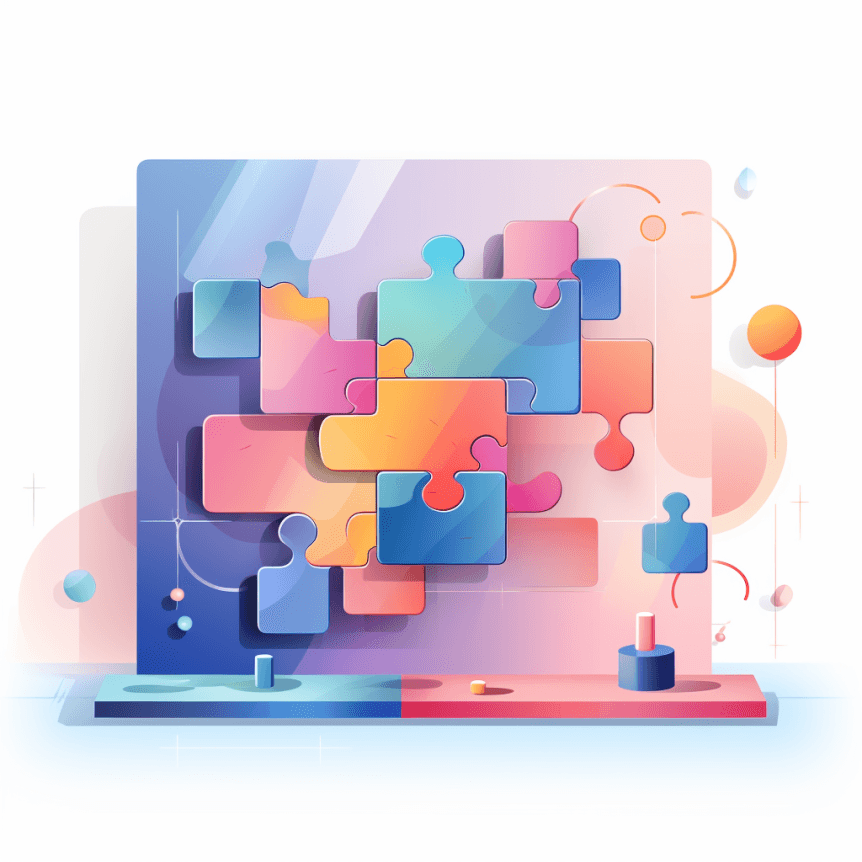
Original Link: https://arxiv.org/abs/2307.15217
Introduction
The introduction of the paper "Open Problems and Fundamental Limitations of Reinforcement Learning from Human Feedback" discusses the potential of Reinforcement Learning from Human Feedback (RLHF) as a promising approach to align AI systems with human values. However, it also highlights the challenges and limitations of RLHF, including the costly and time-consuming process of collecting high-quality data, the need for improved training strategies, and the lack of comprehensive investigation over the effectiveness of human-LLM joint evaluation frameworks. The authors distinguish between challenges that are relatively tractable and could be addressed within the RLHF framework using improved methodology versus ones that are more fundamental limitations of alignment with RLHF. The paper aims to shed light on these challenges and suggest future directions for research in RLHF (Pages 1-5).
Key Insight:
While RLHF presents a promising approach to align AI systems with human values, it comes with significant challenges and limitations that need to be addressed. Distinguishing between tractable challenges and fundamental limitations is crucial for the advancement of RLHF.
Actionable Advice:
For researchers and practitioners working with RLHF, it's essential to understand these challenges and limitations and work towards addressing them. This can be achieved by focusing on effective data collection, improving training strategies, and developing robust evaluation frameworks. Future research should also explore other types of human-in-the-loop solutions to further facilitate RLHF. Additionally, staying updated with the latest research and advancements in RLHF can help in navigating the complexities of aligning AI systems with human values and expectations.

Section 2: Background and Notation
Section 2 provides the necessary background and notation for understanding the challenges and limitations of Reinforcement Learning from Human Feedback (RLHF). It introduces the concept of RLHF and explains how it works, including the process of collecting samples and feedback from humans or groups thereof. The section also discusses the variability of human behavior over time and in different contexts, and how this can affect the RLHF process. It further explains the concept of a rendering function that maps the base model and its examples to what a human sees, which is crucial in the RLHF process (Pages 3-4).
Key Insight:
The variability of human behavior and the way humans perceive the output of the base model are critical factors in the RLHF process. Understanding these factors is essential for effectively implementing RLHF.
Actionable Advice:
For AI startups working with RLHF, it's crucial to understand the variability of human behavior and how humans perceive the output of your model. This understanding can help you design more effective RLHF processes. Consider using techniques such as user testing and feedback collection to gain insights into how different users interact with and perceive your model's output. Also, consider investing in research and development to improve the rendering function of your model, as this can significantly impact the effectiveness of your RLHF process.

Section 3: Open Problems and Limitations of RLHF
Section 3 of the paper "Open Problems and Fundamental Limitations of Reinforcement Learning from Human Feedback" discusses the challenges and limitations associated with RLHF. The authors categorize these challenges into three main types: challenges with obtaining quality human feedback, challenges with learning a good reward model, and challenges with policy optimization.
The authors further distinguish between challenges that are relatively tractable and could be addressed within the RLHF framework using improved methodology versus ones that are more fundamental limitations of alignment with RLHF. The fundamental challenges are substantial enough that overcoming them would require a method that is no longer a form of RLHF. As a result, these fundamental challenges must be either avoided by not using RLHF or compensated for by other safety measures (Pages 4-11).
Key Insight:
The process of RLHF is fraught with challenges, some of which can be addressed within the RLHF framework, while others are more fundamental and may require different approaches or safety measures.
Actionable Advice:
For AI startups working with RLHF, it's crucial to understand these challenges and work towards addressing them. This can involve improving the methodology used within the RLHF framework for more tractable challenges, or considering other safety measures or approaches for more fundamental limitations. Startups should also consider collaborating with the wider scientific community and sharing research findings to collectively address these challenges. Lastly, staying updated with the latest research and advancements in RLHF can help in navigating these complexities.

Addressing Challenges with RLHF
The section titled "Addressing Challenges with RLHF" discusses various methods that can replace or combine with parts of the RLHF pipeline to address challenges associated with human feedback, the reward model, and the policy. The authors present a range of strategies, such as AI assistance, fine-grained feedback, process supervision, translating language to reward, learning from demonstrations, direct human oversight, multi-objective oversight, maintaining uncertainty, aligning LLMs during pretraining, and supervised learning. These strategies are aimed at addressing the various problems with RLHF (Pages 13-15).
Key Insight:
Addressing the challenges with RLHF requires a multifaceted approach that may involve replacing or combining parts of the RLHF pipeline. This includes strategies to improve human feedback, the reward model, and the policy.
Actionable Advice:
For AI startups, it's important to understand that addressing the challenges with RLHF requires a multifaceted approach. This could involve exploring a range of strategies, such as improving the quality of human feedback, refining the reward model, and enhancing the policy. Startups should also consider the potential of combining different parts of the RLHF pipeline to address these challenges more effectively. Lastly, startups should consider collaborating with the wider scientific community and sharing research findings to collectively address these challenges.

Section 4: Incorporating RLHF into a Broader Framework for Safer AI
Section 4 discusses how Reinforcement Learning from Human Feedback (RLHF) can be incorporated into a broader framework for safer AI. The authors argue that while RLHF has clear advantages for aligning AI systems with human goals, it also has limitations and gaps that need to be addressed. They emphasize the need for a multi-faceted approach to the development of safer AI systems, incorporating RLHF into a broader technical safety framework.
The authors suggest that understanding the dynamics between humans and AI, improving the quality of human feedback, and addressing the fundamental problems of AI alignment are crucial for the successful incorporation of RLHF into a broader framework for safer AI. They also highlight the importance of governance and transparency in the development and deployment of AI systems trained with RLHF (Pages 12-17).
Key Insight:
While RLHF is a key component in aligning AI systems with human goals, it should be incorporated into a broader framework for safer AI. This requires understanding the dynamics between humans and AI, improving the quality of human feedback, and addressing the fundamental problems of AI alignment.
Actionable Advice:
For AI startups, it's important to understand that RLHF is not a complete solution for developing safe AI. It should be incorporated into a broader framework that includes other safety measures. Startups should focus on understanding the dynamics between humans and AI, improving the quality of human feedback, and addressing the fundamental problems of AI alignment. They should also prioritize governance and transparency, which could involve implementing auditing and disclosure standards, and working towards improving industry norms and regulations affecting models trained with RLHF.

Section 5: Governance and Transparency
Section 5 discusses the importance of governance and transparency in the implementation of RLHF. The authors argue that a commitment to transparency would make the RLHF research environment more robust from a safety standpoint. They suggest that companies using RLHF to train models for high-stakes or safety-critical applications should maintain transparency with the public and/or auditors about key details of their approach.
The authors also highlight the need for regular reporting of reward components and the ability to compare the capabilities of language models according to standard benchmarks. They acknowledge that incorporating beneficial standards for safety and transparency into norms and regulations affecting AI is an ongoing challenge.
The section also touches on social and economic equity concerns, such as the fair compensation of human subjects used in RLHF research (Pages 15-17).
Key Insight:
Transparency and governance are crucial for the safe and ethical implementation of RLHF. This includes transparency about the details of RLHF implementation, regular reporting, and fair compensation for human subjects.
Actionable Advice:
For AI startups, it's important to prioritize transparency and governance in your RLHF implementation. This could involve disclosing key details of your RLHF approach, regularly reporting on reward components, and ensuring fair compensation for human subjects. Additionally, startups should stay updated with norms and regulations affecting AI and work towards incorporating beneficial safety and transparency standards into their practices. Lastly, consider collaborating with the wider scientific community and sharing research findings to collectively address these challenges.

Section 6: Discussion
Section 6 of the paper discusses the fundamental challenges with RLHF. The authors note that while technical progress in some areas is tractable and should be seen as a cause for concerted work and optimism, other problems with RLHF are fundamental. Overcoming these would require a method that is no longer a form of RLHF. As a result, these fundamental challenges must be either avoided by not using RLHF or compensated for by other safety measures. The authors also note that many of the problems RLHF faces are not new and represent broader challenges in ML (Pages 17-18).
Key Insight:
The challenges with RLHF are not unique and represent broader challenges in machine learning. Addressing these challenges requires a combination of improved methodologies, alternative approaches, and additional safety measures.
Actionable Advice:
For AI startups, it's important to understand that while RLHF is a promising approach, it comes with fundamental challenges that may require alternative methods or additional safety measures. Startups should consider adopting a multi-faceted approach to AI safety, incorporating RLHF along with other safety measures. This could involve exploring alternative methods, improving existing methodologies, and implementing additional safety measures. Startups should also consider collaborating with the wider scientific community and sharing research findings to collectively address these challenges. Lastly, staying updated with the latest research and advancements in RLHF and broader ML challenges can help in navigating these complexities.